Today we're talking about RxJava, the library that implements the ideas of the reactive extensions for composing asynchronous and event-based programs using observable sequences. Along the way, we look at basic RxJava classes, observables, and data processing functions, before ending with a link to our one-page RxJava cheat sheet PDF.
What Is RxJava?
RxJava is an implementation of the reactive extensions, a library for composing asynchronous and event-based programs using observable sequences.
As such, RxJava offers the implementations of the abstractions to create streams of events and items that you can subscribe to in your code, and react to the data flowing through those streams. Note, these are different from the streams you may be familiar with in the Java 8 Streams API.
Basic RxJava Classes
Here are the basic RxJava classes you should know about:
Observable
emits 0 or n items of T and terminates with complete or an error.Flowable
is an addition that came in RxJava 2. It acts as an Observable, and emits 0 or n items. It terminates with complete or an error, but it also supports back pressure, which lets you control how fast the source emits items. It's really handy when you're building complex systems, since a fast producer of data cannot flood the system with items waiting to be processed.Single
, an Observable, which either emits a single item or throws an error. Think of it as the reactive version of a method call. It's essentially a performance optimization of Observable — when you know that only a single item can pass through, you might cut some corners in the implementation and drop some checks to make it work faster.Maybe
succeeds with either an item, no item, or errors. It's the reactive version of an Optional.Completable
either completes or returns an error. It never return items. Think of it as a reactive version of a Runnable.
There are more classes in the API you need to know, but these are responsible for creating the streams and producing the data. On the other side, data is processed via Observers. We'll talk about them in a moment.
Back to topObservables in RxJava
Most of the time in a system, you'll have some way to get the observables from the API. Here's how you can create an observable from a value; just call it the just
method. There's also a way to create an Observable from a collection, which will emit all the items in the collection one by one and allow the observers to have a chance to process them.
Observable.just("RebelLabs"); Observable.fromIterable(iterable); Observable.fromCallable(callable);
The attentive reader might have nodded knowingly at our third example in the snippet above, the one where you can create an Observable from a callable. This comes really handy when you're building an asynchronous API.
Subscribing to Observables
Now that we have the building blocks to create reactive streams of data, the missing piece is processing the data that flows through them. This is exactly what Observers allow you to do. Observers provide a mechanism for receiving data and notifications from Observables using the following API:
onNext(T t)
provides the Observer with a new item to observe.onError(Throwable e)
notifies the Observer that the Observable has experienced an error condition.onComplete()
notifies the Observer that the Observable has finished sending push-based notifications.
To subscribe to the observables, you just implement the Observer interface and put your business logic into the onNext
method while using the other two to manage the lifecycle notifications from the observable.
Publishing to Multiple Observers in RxJava
Sometimes, you want to have more than a single Observer being notified of the items emitted from an observable. For example, imagine that you're building a UI and need to process user actions. You might want to log the action, start a computation, or change the UI to convey that an operation is in progress. All these actions are independent and it'd be smart not to mix them up with business logic. So, you'd want multiple Observers to receive an item saying something happened, so the logger would log, the UI updater would update the UI, and so on.
Luckily, there's a way to do that. You'll need to use publish()
to subscribe multiple Observers to an Observable. With that, the Observable would be able to receive multiple Observers and won't emit any items until you call the connect
method. Then, it'll start notifying all the Observers of the items.
Observable topic = Observable.publish().autoConnect(2); topic.subscribe(subscriber1); topic.subscribe(subscriber2);
You can also use the autoConnect(int)
method to say how many Observers you're going to connect. This way, you won't need to call the connect method manually and the items will flow automatically when all the Observers are attached.
Back to topWant to see what tools Java developers are using in 2020? Check out our latest Java Productivity Report! You can watch a breakdown of the results via the video below, or download the full report for free by clicking here.
Data Processing Functions in RxJava
In this section, we look at the most frequently used functions used to process the data flowing through the Observables. By applying these on an Observable, you'll get another Observable which you can subscribe to.
You can recognize some of these from the Java 8 Stream API since most stream processing libraries have the basic functions that are very similar.
map(Function<? super T,? extends R> mapper)
applies a function to each of the items, and emits the returned values.filter(Predicate<? super T> predicate)
emits only the items satisfying a predicate.buffer(int count)
emits lists of the items of the specified size.zip(ObservableSource s1, ObservableSource s2, BiFunction<T1, T2, R> f)
applies a function to the items from multiple observables and emits the returned value.flatMap(Function<? super T,? extends ObservableSource<? extends R>> mapper)
takes a function from items to an Observable, emits the items of the resulting ObservablesgroupBy(Function<? super T,? extends K> keySelector)
emits items grouped by a specified key selector functiontimeout(long timeout, TimeUnit timeUnit)
emits items of the original observable. If the next item isn't emitted within the specified timeout, a TimeoutException occurs.
RxJava documentation comes with the marble diagrams — pictures that illustrate what happens with the data when you apply a function. They look amazing and are quite intuitive. In the cheat sheet, we illustrated the flatmap behavior with a marble diagram:
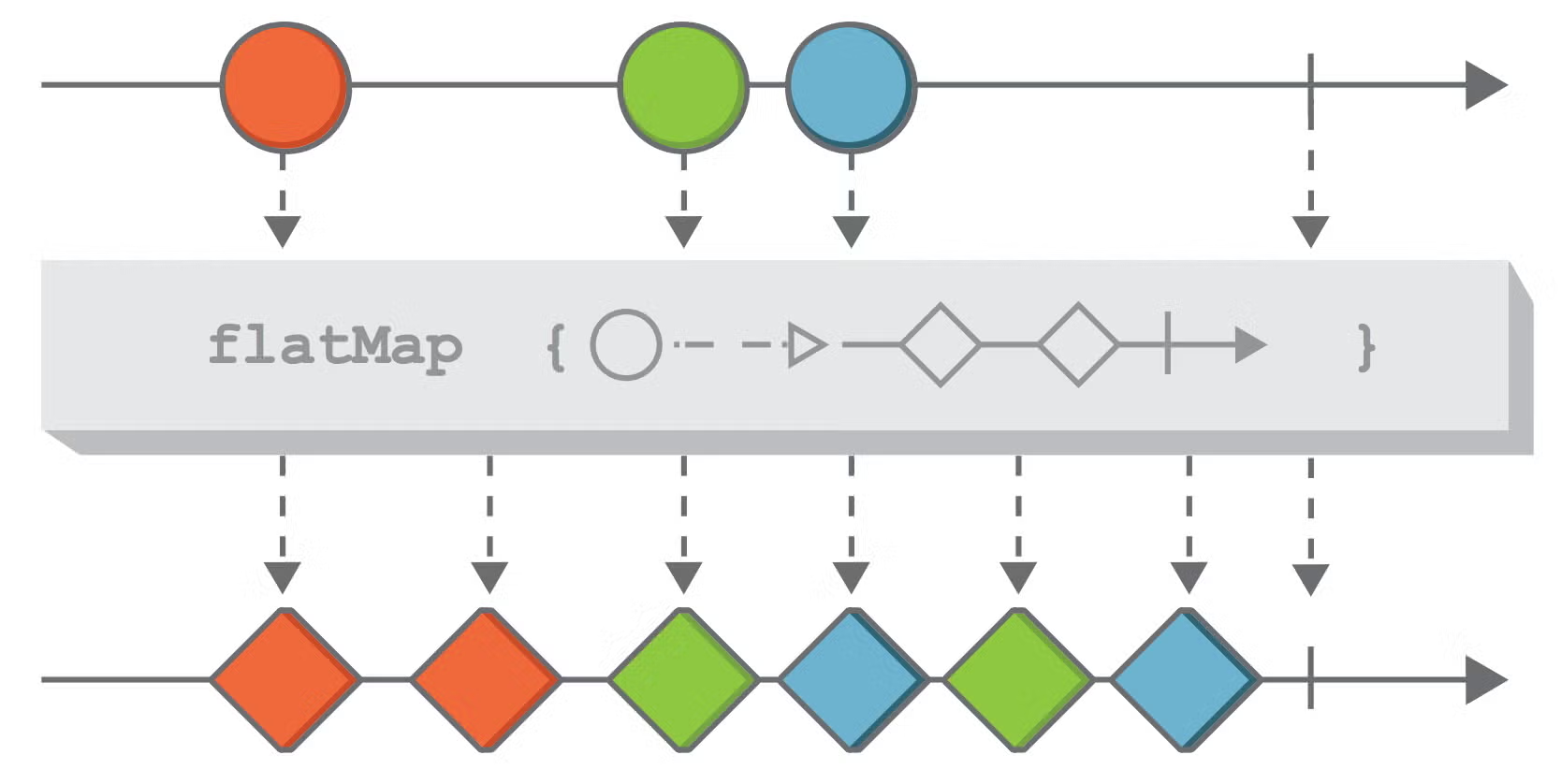
Testing Observables in RxJava
A great thing about RxJava is that it's production ready and developer friendly. For example, they have a way to test the Observables and the data transformation functions you use.
TestSubscriber
is a subscriber that records events that you can make assertions upon.TestObserver
is an Observer that records events that you can make assertions upon.
Here's a basic example of using a TestSubscriber to check if the range
function creates an Observable that emits N items.
TestSubscriber ts = Flowable.range(1, 5).test(); // assert properties of the flow assertThat(ts.values()).hasSize(5);
Next, let's look at some of the libraries that can help you use RxJava on Android. One reason we're interested in using RxJava on Android is because ZeroTurnaround develops JRebel for Android, an Android Studio plugin that skips the build and install cycle when developing, and allows you to simply reload your Android application code right in the device or emulator on the fly.
Back to topRxLifecycle
One problem with RxJava on the Android platform is the complicated lifecycle of the Android framework components. Activities and fragments can come and go as the framework pleases, and that means you always have to be sure you clean up all the resources that you attached to them. In the Rx world, it's a bit more complicated, since you don't build the graph of objects in the normal way, but you instead attach the data processing functions in the reverse order. So, either you always cancel the subscriptions manually or use something like the RxLifecycle library.
RxLifecycle allows you to bind subscription lifecycles to the lifecycle of the Android components. It will automatically destroy the subscriptions and avoid memory leaks when the Android component is destroyed.
myObservable.compose( RxLifecycleAndroid.bindActivity(lifecycle)).subscribe();
Just compose your Observable and call one static method from the library — it doesn't get much easier. There's also a more verbose API to manually specify when to cancel the subscription; but for most cases, you're good with the default one.
RxBinding
One very Android-specific use case for RxJava is managing UI events and actions with Observables. To simplify doing it, RxBinding offers a API to unify all the UI event handlers.
You can use the RxView
class to turn UI events into RxJava Observables:
Button button = (Button) findViewById(R.id.button); RxView.clicks(button).subscribe(x -> { // do work here });
This will work the same way for all the UI elements: buttons, text views, and so on.
RxAndroid
Another thing you should be careful of when developing Android apps is on which thread to process the data. You cannot do any long running computations on the UI thread, as it'll render the UI unresponsive. Instead, you want your Observers to operate on background threads. To make it seamless, use RxAndoid library, which you can easily control which threads you subscribe to Observables.
Observable.just("RebelLabs") .subscribeOn(Schedulers.newThread()) .observeOn(AndroidSchedulers.mainThread()) .subscribe(/* an Observer */);
In the snippet above, we’re observing the events on the main Android looper thread, and the subscription actions will be executed on the new background thread. The library offers more customizations, but for the most part, the defaults will work nicely.
Whenever you use RxJava, you'll use Observables, Observers, and transform the data, and this cheat sheet can be a great reminder of the most frequently used parts of Rx Java.
Back to topDownload the RxJava Cheat Sheet PDF
Download and print out this cheat sheet so you can use it whenever you need. For greater explanations and detailed content in the cheat sheet, bookmark this blog post!
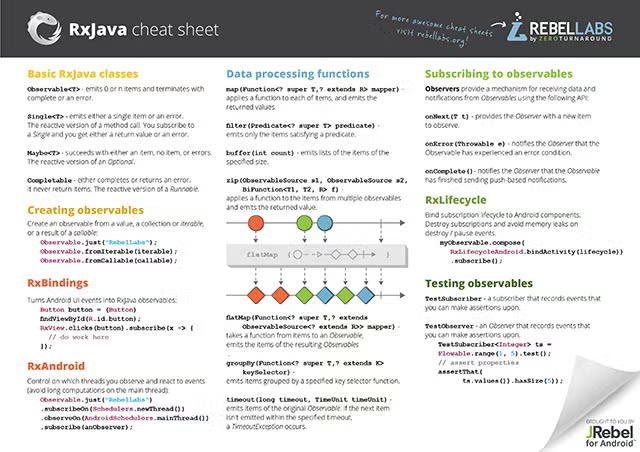
Additional Resources
Ever since Anton wrote a post about RxJava, I wanted to create an RxJava cheat sheet. With RxJava API, data processing functions, some lesser known tricks and utilities, we have the perfect mix for a great cheat sheet — and trust me, we've created a bunch of them. Here's a selection of our most popular ones:
And many more. You can see all of them on our cheat sheets page.
Want to Save Time During Development?
JRebel can help developers skip redeploys and instantly see changes to code. Want to try it on your project? Click the link below to learn more about our free JRebel trial.